2x more risk, 25% less depth: Meet the super-respondent. 2x more risk, 25% less depth: Meet the super-respondent.
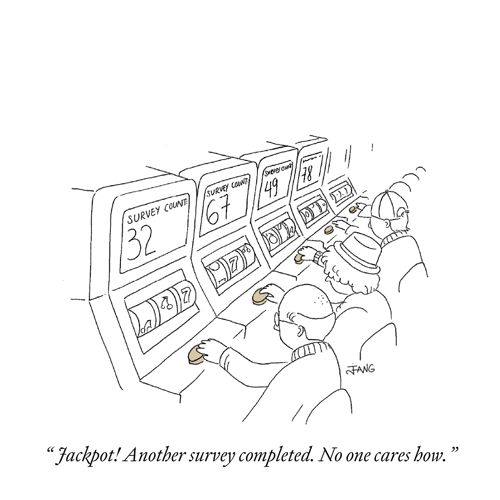
- Reading time
- 1 min
- Words
- Adrien Vermeirsch
- Published date
- May 13, 2025
Would you take 25 surveys in one day? What about 50, or even 100? Probably not. Most people wouldn’t. But when you commission survey research, that’s exactly the kind of behavior that might be quietly distorting your results. Our new research measures the hidden threat of “super-respondents”.
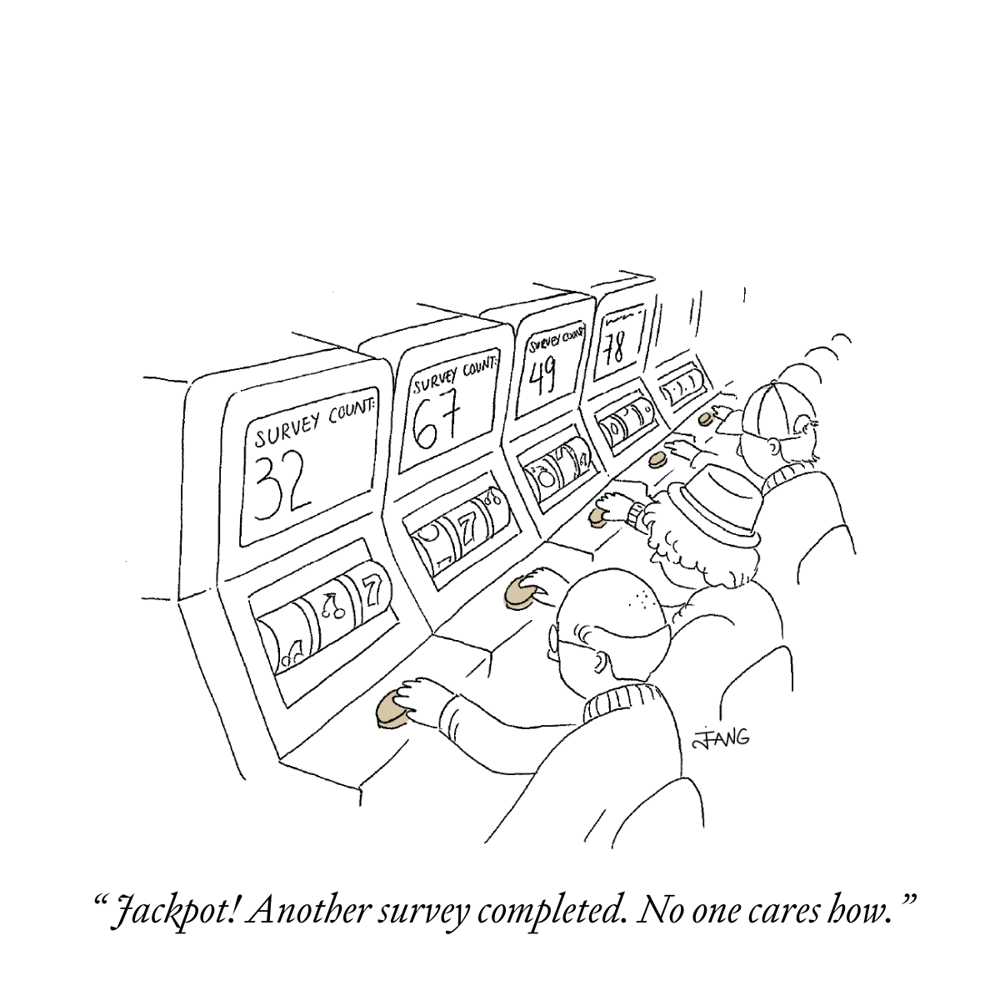
Is your strategy being built on someone’s 100th survey of the day?
Whether it’s for market sizing or due diligence, market research helps strategists in consulting and private equity reach the consumers and professionals who matter most.
But when you launch an online survey, it doesn’t just reach your target audience. It enters a crowded, incentive-driven ecosystem where speed and volume are rewarded. Some respondents are thoughtful, others are clearly fraudulent, and then there are those in between: people who’ve taken 30, 60, or even 100 surveys before taking yours.
We call them super-respondents.
Super-respondents are a mixed bag. Some are real people trying to earn money by completing as many surveys as possible. Others are bots or click farm accounts designed to mimic legitimate behavior. Some get flagged by fraud detection tools, but many pass off as “clean” data.
Fraud isn’t the throughline — it’s volume. And that volume can affect your insights. Even when super-respondents pass your providers’ data quality checks, they’re more likely to skim, rush, and follow the most predictable answering patterns in your survey.
Most importantly, because super-respondents help vendors hit survey quotas faster, many treat them as valid completes — passing them off as quality data.
What providers don’t tell you can hurt you.
Because of the black-box nature of the survey industry, most buyers never see what’s behind their sample, even when that data can hurt strategic decisions, client relationships, or investment returns.
Survey frequency (how many surveys a person attempts in the last 24 hours) is one of the most tangible metrics to gauge data quality and respondent engagement. And while some providers do apply limits on survey attempts as a data quality measure, thresholds vary and are not disclosed to survey buyers.
There’s also little evidence around where the cutoffs should be: how many surveys is too many?
That’s what we set out to test.
Our study.
Building on Potloc’s first research on research study about the importance of sample sources, and a second one on the impact of survey design, we ran a third investigation focused on survey frequency.
We surveyed 3,000 U.S. adults across five different sample sources, testing how the volume of survey attempts in the last 24 hours impacts data quality, depth of responses, and research feasibility. To do this, we removed our normal survey frequency limits (i.e., no more than 30 survey attempts in the last 24 hours) and let all survey entrants through, regardless of how many surveys they’d already taken that day.
All participants were subjected to the same pre-, during, and post-survey data quality controls, and we tracked survey frequency using Research Defender’s Activity Score, which measures how many surveys a person has attempted in the last 24 hours.
We classified survey participants into 4 different categories based on their survey frequency:
Less than 5 survey attempts in the last day. Passed pre-, in-, and post-survey quality checks.
Between 5 to 30 survey attempts in the last day. Real people taking multiple surveys per day. Not always low quality — but at higher risk of fatigue.
Between 31 to 100 survey attempts in the last day. Super-respondents, often treated as "clean" by vendors in order to hit target, but highly likely to distort results through speed, habit, or disengagement.
We then analyzed key variables such as respondent classification, quality checks at each stage (pre-, in-, and post-survey), and length of interview across the four frequency bands. This gave us a clean state to observe:
- How often do super-respondents fail quality checks?
- How much does their behavior differ (engagement, depth of response, time spent)?
- What do survey buyers stand to lose — or gain — by tightening frequency thresholds?
Key results.
At a glance.
x2
more bad quality.
Super-respondents were twice as likely to fail data quality checks.
25%
less time spent.
Even when passing data quality controls, super-respondents rushed through surveys more.
<30
survey attempts.
Data quality starts to degrade sharply after 30 survey attempts in 24 hours.
1. More survey attempts meant more bad data.
The correlation was clear: the more surveys someone had taken in the past day, the more likely they were to fail our quality checks. Among casual survey takers, just 11% were flagged as poor quality. For those who had attempted more than 30 surveys, that number doubled.
This pattern held true across every phase of our data checkpoints — pre, during, and post-survey data quality controls. Super-respondents were more likely to be inconsistent, disengaged, or flagged for unusual response patterns.
Takeaway
Survey frequency is a hidden variable with massive consequences, especially when buyers are left in the dark about whether and how providers filter it out.
2. Super-respondents’ answers didn’t match those of casual respondents.
Even when the data passed validation, their responses weren’t the same as casual respondents. In 90% of questions analyzed, the distribution of responses changed based on how many surveys the participant had attempted that day.
In one question, we intentionally limited the multiple-choice options to see if respondents would take the time to write in a custom answer under “Other.” Casual respondents were much more likely to do so, while professional survey takers overwhelmingly selected a default response, despite it not being a true fit.
Takeaway
This suggests that higher-frequency respondents, even when not purely fraudulent, still impact your data by being conditioned to skim, speed through, or game the logic.
3. Super-respondents provided shorter, less engaged responses.
We also looked at the quality of open-ended responses. Median length of open-ended questions and time spent on the survey was significantly lower for higher-frequency groups: Casual respondents gave longer, more detailed answers on average. They also spent more time taking the survey than super-respondents and pure fraud/bots, spending a median of 9.3 minutes (versus 6.9 minutes for super-respondents). This gap represents a 25% drop in survey length.
Takeaway
Even when they weren’t purely fraudulent, super respondents moved fast and offered less depth and thoughtfulness in their responses. For consulting and PE firms relying on qualitative insights or customer voice analysis, this engagement disparity can weaken the power of their data.
Conclusions.
Our findings show that not all “clean” data is created equal. Higher-frequency respondents may pass fraud checks, but their impact still shows up in:
- The shape of your response distributions.
- The total time they’re willing to spend thinking through your questions.
- Subtler insights, like depth in open-ended responses.
In high-stakes research, these details matter. Especially when your goal isn’t just to spot trends, but to understand deeper nuances.
Moreover, these respondents aren’t rare. In many panels and marketplaces, they are the norm. The system is optimized to reward volume over depth. Unless you’re asking hard questions about source quality, respondent experience, or frequency thresholds, it’s easy for professional survey takers to slip through for the benefit of providers hitting their quotas faster and for cheaper.
Most survey buyers never see the breakdown. Providers rarely share how many respondents were excluded or what thresholds were used to clean the data. The final dataset may look tidy, but here’s what’s typically left out:
- How many respondents were excluded, and why.
- Whether higher-frequency respondents were filtered (or not).
- What quality thresholds were applied.
- How much of your sample came from good, casual survey takers.
Survey design tips for consulting & PE firms.
1. Understand that high-quality respondents come with trade-offs.
The more surveys someone attempts in a day, the more likely their responses are to underperform. However, we also found that while stricter survey frequency cutoffs increase data quality, they greatly reduce the potential respondent pool. It’s a practical balance between quality and feasibility.
At Potloc, we cap daily attempts at 30 per respondent, because that’s where we start to see data degradation climb (For context, RepData, the leading third party providing us this data, recommends a threshold of 75.)
Your data source matters too — not all survey methodologies deliver the same mix of respondent types. Potloc’s proprietary social media sampling technology and our own respondent community, for example, yielded respondents with lower survey frequencies.
Find a survey partner who can help you manage these sampling and data cleaning complexities based on your risk tolerance and project priorities.
2. Demand more transparency from your survey providers.
In your next survey, start asking the questions that impact your research outcomes, ROI, and client relationships.
Most buyers don’t know what frequency thresholds were applied, if any. They don’t know how many respondents were excluded or what platforms those respondents came from. And they almost never see breakdowns by sample source or respondent type.
If your provider can’t (or won’t) answer such questions clearly, think about what they’re hiding.
3. Address the root cause of bad data.
Fraud detection is critical, and it’s come a long way as technology has advanced. But it only treats part of the problem. Today’s survey system still makes it easier to game than to engage. Low incentives, clunky survey design, and poor targeting prioritize volume and push thoughtful respondents out and reward the fastest fingers.
Respondents pretty much have two options:
- Try a few surveys, make less than 75 cents in an hour, and never come back.
- Learn how to game the system through volume.
As a buyer, you help shape this system. Designing better surveys — or working with providers who do — attracts the kind of respondents your research deserves. It's not just about removing fraud. It’s about shifting the ratio by attracting more honest, engaged people.
Transparency,
built in.
For consulting and PE firms, data quality isn’t just a checkbox. It’s a risk factor.
Potloc’s platform gives you the transparency you deserve for every project: where your respondents came from, which data quality controls they passed, and key metrics like good-to-bad ratios and survey attempts. No more “mystery samples” — get the context you need to trust your data.